Scientometrics Landscapes
This post is to introduce a technique for the exploration of the scientific literature on which the médialab has been working intensively in the last few months.
Post
By Tommaso Venturini
This post is to introduce
on which the médialab has been working intensively in the last few months. This technique aims at extracting a
in a given corpus of bibliographical notices.I am writing this post with two main objectives:
- 1) Providing the clearest possible explanation of a complex method that I’ve introduced to many (but that I have succeeded in explaining only to few).
- 2) Luring developers in search for a cool project to help us out on this technique. As I’ll explain there are already tools the implement it, but they need improvement.
To provide some context, we have developed this technique in the framework of the project Politiques de la Terre, in order to compare the different meanings of
. Mainly because of its contribution to the climate crises, this humble chemical molecule has recently become one of the most important actors of our collective life. Carbon dioxide is increasingly used as a key marker for national and international policies and has assumed a variety of meanings according to who invoke its name. Chemists, biologists, geologists, soil scientists, physicists, climatologists, they all have different CO2 definitions. And their definitions differ from those of the economists, geo-politicians and NGOs and probably even more from the different representations that public opinion may have of the molecule.
. As a example of what this technique can deliver, see below the scientometrics landscapes obtained in the CO2 project for the period 2001-2006
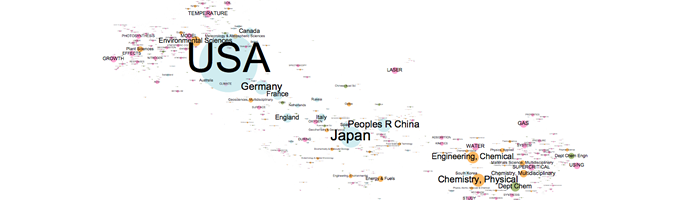
Click on the image to enlargeThe procedure followed to obtain this figure is a 7-steps recipe:
- 1) Find a query capturing in a bibliographic database (ISI Web of Science, for example) as much as possible of the scientific literature on given subject, with the minimum possible noise (e.g. all papers on CO2 and only them).
- 2) Download a relatively large corpus of bibliographical records (at least 2.000, but we have almost 300 thousands in the CO2 project) with all the attached metadata.
- 3) Extract all the references cited in the bibliographies of such notices.
- 4) Construct a network whose nodes are the extracted references linked by appearing in the bibliographies of the same articles (since Small-1973 co-citation is known to be a efficient way to highlight disciplinary cohesion).
- 5) Spatialize the reference network with a force-vector algorithm (we recommend ForceAtlas2 of course – Jacomy et al, 2014) to reveal disciplinary and sub-disciplinary clusters and then block the position of the references. This will be your background map.
- 6) Extract all the available metadata (authors, keywords, subject categories, institutions, countries…) from your corpus of bibliographical records and project them on the references background map. Connecting each meta-item to the references cited in the paper that contains that meta-item. Do not connect metadata among themselves, but only to references. This is tricky, so here is an example: if your corpus contains a bibliographical notice wrote by “P. Girard” at the “Sciences Po médialab” using the keyword “datascape navigation” and citing “Latour, 2005”, in your network “P. Girard”, “Sciences Po médialab” and “datascape navigation” will all be connected to “Latour, 2005” (but not among themselves).
- 7) Spatialize the metadata while keeping the references blocked (so that that the metadata positions themselves relatively to the references landscape).
Here a couple of tricks to make your results more interesting:
- 1) Clean manually your metadata between step 6 and 7 (e.g. if you used the keyword CO2 in your query, it will be by definition very present in the map so it is not very interesting to keep it).
- 2) Generate different maps for different temporal periods.
This sounds complicated and it definitely is, but there’s good news: the médialab has already worked on two tools that will accompany you through many of the steps listed above.The first tool,
, has been developed by Mathieu Jacomy as a module of
(http://tools.medialab.sciences-po.fr/sciencescape/scopus_referencescape.php). This module is intended as a tool for students and people with no coding skills. It does automatically everything described in step 3 to 7. The tool is incredibly easy to use – just upload a Scopus extraction and wait for results to pop-up (but be patient because it may take up to 15-20 min depending on the size of your corpus and the computation resources of your computer).Here is a video driving you through the usage of this tool:
Iframe https://player.vimeo.com/video/113290065
ReferenceScape is a great quick&dirty exploration tool, but its lack of personalization hinders sophisticated analysis. Users searching for something more advanced can try
(https://github.com/medialab/bibliotools3.0/). BiblioTools has been initially developed by Sebastian Grauwin (http://www.sebastian-grauwin.com/?page_id=427), under the supervision of Pablo Jensen (see Grawin & Jensen 2011, and Grawin et al., 2012), and then heavily optimized by Paul Girard.BiblioTools is already a great tool (the images discussed above are produced with it), but it could be made even greater with some improvement (https://github.com/medialab/bibliotools3.0/issues). The médialab and Sebastian Grauwin will keep working on this project in the future, but if there are keen developers out there, please get in touch.PS.There is something that I have omitted in the text above and it is the crucial important of thresholds. The method of scientometrics landscape works better on large corpora of bibliographical notices (let’s say more than 10.000), because a higher number of co-citations produce more reliable epistemic clustering. The problem with large corpora, however, is that the also contains thousands (hundreds of thousands) of metadata-items producing networks that are too rich to be legible (and even too big to be opened in Gephi). Thus the need to filter most of the metadata (and some of the references). And this is where the magic happens or, in most cases, where the magic fails to happen.Filter too much and you’ll lose all the interesting nodes (e.g. the authors that bridge different epistemic communities; the boundary keywords that allow interdisciplinarity; the highly specialized journals where sciences is actually innovated). Filter too little and you’ll have an unmanageable network. It also gets more complicated because there are at least two variables on which you can filter (there is certainly more, but this tools are implemented in the tools above): the number of occurrence of nodes (the number of bibliographical notices in which a reference or a metadata appears) and the weight of edges (the number of bibliographical notices in which a reference
a metadata appear together). Unfortunately, I have no trick to suggest here. The only advice that I can give is: do not trust the first result that you get, try varying the combination of thresholds until you obtain interesting and robust results.
References
Jacomy, M., Venturini, T., Heymann, S., & Bastian, M. (2014). ForceAtlas2, a Continuous Graph Layout Algorithm for Handy Network Visualization Designed for the Gephi Software. PloS One, 9(6), e98679. doi:10.1371/journal.pone.0098679Small, H. (1973). Co-citation in the scientific literature: A new measure of the relationship between two documents.
,
, 265–269.Grauwin, S., Jensen, P. (2011). Mapping Scientific Institutions. Scientometrics 89(3), 943-954 Grauwin, S., Beslon, G., Fleury, E., Franceschelli, S., Robardet, C., Rouquier, JB., Jensen, P. (2012). Complex systems science: dreams of universality, reality of interdisciplinarity. Journal of the American Society for Information Science and Technology, ASIS&T 63(7):1327-1338.